报告题目:超越深度强化学习:网络优化中的生成扩散模型(GDM)教程
报告人:Dusit Niyato教授
时间:2024年3月5日 10:00-11:00
地点:友谊校区正禾宾馆东三会议室
邀请人:刘家佳教授
报告简介:生成扩散模型(Generative Diffusion Models, GDMs)已在生成人工智能(Generative Artificial Intelligence, GAI)领域崭露头角,展示了其在各种应用中的多功能性和效能。GDMs 能够建模复杂的数据分布并生成高质量的样本,在图像生成和强化学习等任务中表现出特别的效果。此外,它们的迭代性质——涉及一系列的噪声添加和去噪步骤——是一种在学习和生成数据方面强大而独特的方法。本演讲介绍了在网络优化任务中应用 GDMs 的方法。我们深入探讨了 GDMs 的优点,强调了它们在各种领域的广泛适用性。本演讲提供了 GDMs 及其在网络优化中的应用的基本背景,以及一系列具体案例研究,展示了 GDMs 与深度强化学习(Deep Reinforcement Learning, DRL)、语义通信(Semantic Communications, SemCom)和车联网(Internet of Vehicles, IoV)网络的集成。这些案例研究强调了 GDMs 在现实场景中的实用性和效能,为网络优化与设计提供了洞见。
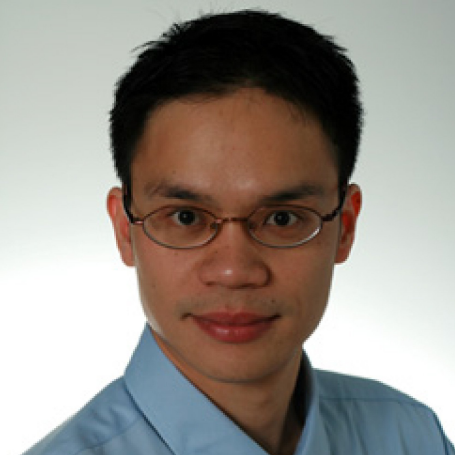
Title: Beyond Deep Reinforcement Learning: A Tutorial on Generative Diffusion Models (GDMs) in Network Optimization
Prof. Dusit Niyato
Nanyang Technological University
Abstract:
Generative Diffusion Models (GDMs) have emerged as a transformative force in the realm of Generative Artificial Intelligence (GAI), demonstrating their versatility and efficacy across a variety of applications. The ability to model complex data distributions and generate high-quality samples has made GDMs particularly effective in tasks such as image generation and reinforcement learning. Furthermore, their iterative nature, which involves a series of noise addition and denoising steps, is a powerful and unique approach to learning and generating data. This presentation gives an introduction on applying GDMs in network optimization tasks. We delve into the strengths of GDMs, emphasizing their wide applicability across various domains. The presentation first provides a basic background of GDMs and their applications in network optimization. This is followed by a series of case studies, showcasing the integration of GDMs with Deep Reinforcement Learning (DRL), Semantic Communications (SemCom), and Internet of Vehicles (IoV) networks. These case studies underscore the practicality and efficacy of GDMs in real-world scenarios, offering insights into network design.
Biography:
Dusit Niyato is currently a President's Chair Professor in Computer Science and Engineering in the College of Computing & Data Science (CCDS), Nanyang Technological University, Singapore. He received B.E. from King Mongkuk’s Institute of Technology Ladkrabang (KMITL), Thailand in 1999 and Ph.D. in Electrical and Computer Engineering from the University of Manitoba, Canada in 2008. Dusit's research interests are in the areas of distributed collaborative machine learning, Internet of Things (IoT), edge intelligent generative AI and AI-generated content (AIGC), mobile and distributed computing, and wireless networks. Dusit won the IEEE Communications Society (ComSoc) Best Survey Paper Award, IEEE Asia-Pacific Board (APB) Outstanding Paper Award, the IEEE Computer Society Middle Career Researcher Award for Excellence in Scalable Computing and Distinguished Technical Achievement Recognition Award of IEEE ComSoc Technical Committee on Green Communications and Computing. Currently, Dusit is serving as Editor-in-Chief of IEEE Communications Surveys and Tutorials (impact factor of 35.6 for 2023), an area editor of IEEE Transactions on Vehicular Technology, editor of IEEE Transactions on Wireless Communications, associate editor of IEEE Internet of Things Journal, IEEE Transactions on Mobile Computing, IEEE Wireless Communications, IEEE Network, IEEE Transactions on Information Forensics and Security (TIFS), and ACM Computing Surveys. He was a guest editor of IEEE Journal on Selected Areas on Communications. He is the Members-at-Large to the Board of Governors of IEEE Communications Society for 2024-2026, and was a Distinguished Lecturer of the IEEE Communications Society for 2016-2017. He was named the 2017-2023 highly cited researcher in computer science. He is a Fellow of IEEE and a Fellow of IET.